Accelerating Materials Discovery
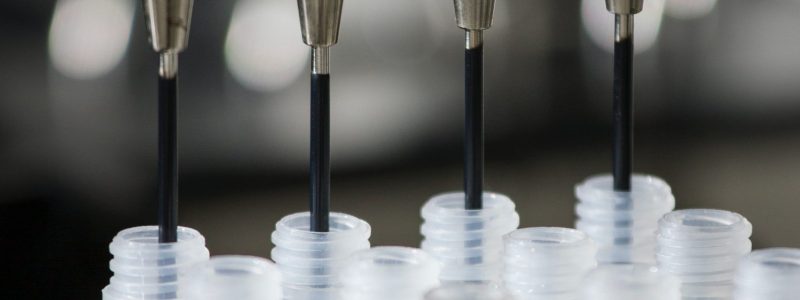
Traditional research methods—in which a researcher sequentially optimizes a synthesis procedure to make a new material, one at a time—limits the number of experiments to of order 50 per month or less. As laboratory robotics improve and become more accessible, researchers can perform experiments at an increased rate. New advances in parallel experimentation have increased this number to 10s to 100s of synthetic experiments per day. A3MD is partnered with the National Research Council of Canada (NRC) to host the infrastructure required to perform rapid, autonomous, parallelized synthetic experimentation.
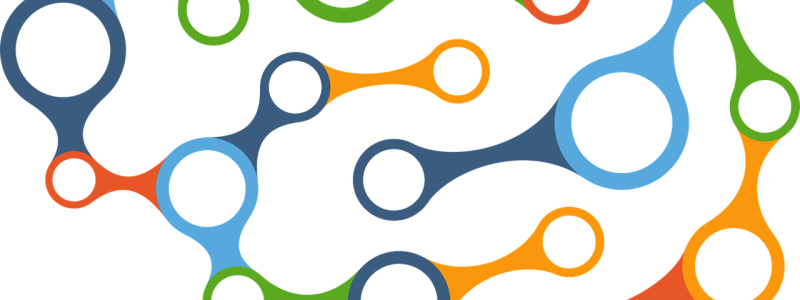
In conventional materials development, humans use the data output from one experiment to inform the design of subsequent experiments. This becomes increasingly ineffective as the amount of data output rises exponentially through accelerated development, and human pattern recognition is ill-suited to highly multi-dimensional decision making. The A3MD model is to use machine learning techniques such as Bayesian Inference to learn complex trends (that would not be obvious to human researchers) in high-order data output space to guide future experiments.
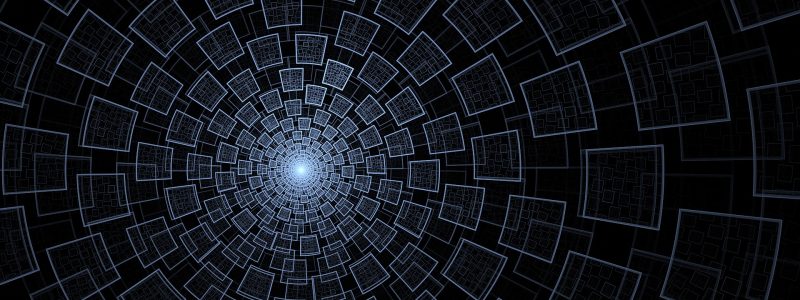
Conventional materials discovery starts with first-principle calculations to explore the available chemical space of a new class of materials. These calculations, such as those based on density functional theory, are computationally expensive. This greatly limits the number of different material combinations that can practically be studied. The A3MD model is to train machine learning models on a small number of calculated materials, which is then used to rapidly explore a much larger set. This acceleration enables the screening of several orders-of-magnitude more compounds than if conventional first-principle methods are used alone, and at a similar accuracy.
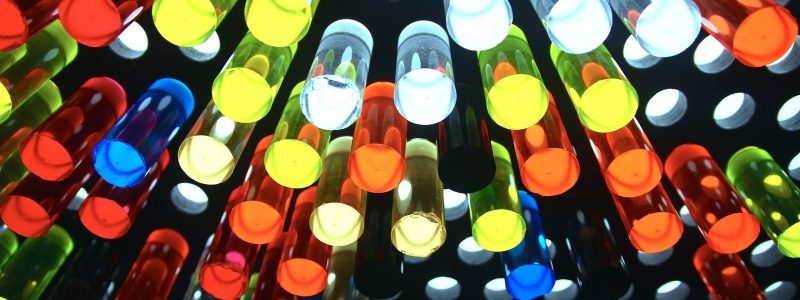
The evaluation of the performance of materials is often the bottleneck in the discovery process; and can eliminate much of the benefit gained from accelerating the first two steps. A3MD places an equal emphasis on rapidly testing the performance of synthesized materials. Where accelerated evaluation of a certain metric is impossible, machine learning will be leveraged to learn proxies for the desired metrics.
Sample Publications
Representative accelerated materials discovery publications from our academic teams. Check back soon for A3MD's early publications.
Accelerated discovery of CO2 electrocatalysts using active machine learning
M. Zhong, K. Tran, Y. Min, C. Wang, Z. Wang, C. T. Dinh, P. De Luna, Z. Yu, A. S. Rasouli, P. Brodersen, S. Sun, O. Voznyy, C. S. Tan, M. Askerka, F. Che, M. Liu, A. Seifitokaldani, Y. Pang, S. C. Lo, A. Ip, Z. Ulissi, E. H. Sargent
Link to Publication Click for AbstractThe rapid increase in global energy demand and the need to replace carbon dioxide (CO2)-emitting fossil fuels with renewable sources have driven interest in chemical storage of intermittent solar and wind energy. Particularly attractive is the electrochemical reduction of CO2 to chemical feedstocks, which uses both CO2 and renewable energy. Copper has been the predominant electrocatalyst for this reaction when aiming for more valuable multi-carbon products, and process improvements have been particularly notable when targeting ethylene. However, the energy efficiency and productivity (current density) achieved so far still fall below the values required to produce ethylene at cost-competitive prices. Here we describe Cu-Al electrocatalysts, identified using density functional theory calculations in combination with active machine learning, that efficiently reduce CO2 to ethylene with the highest Faradaic efficiency reported so far. This Faradaic efficiency of over 80 per cent (compared to about 66 per cent for pure Cu) is achieved at a current density of 400 milliamperes per square centimetre (at 1.5 volts versus a reversible hydrogen electrode) and a cathodic-side (half-cell) ethylene power conversion efficiency of 55 ± 2 per cent at 150 milliamperes per square centimetre. We perform computational studies that suggest that the Cu-Al alloys provide multiple sites and surface orientations with near-optimal CO binding for both efficient and selective CO2 reduction. Furthermore, in situ X-ray absorption measurements reveal that Cu and Al enable a favourable Cu coordination environment that enhances C–C dimerization. These findings illustrate the value of computation and machine learning in guiding the experimental exploration of multi-metallic systems that go beyond the limitations of conventional single-metal electrocatalysts.
Crystal site feature embedding enables exploration of large chemical spaces
H. Choubisa, M. Askerka, K. Ryczko, O. Voznyy, K. Mills, I. Tamblyn, E. H. Sargent
Link to Publication Click for Abstract
Mapping materials science problems onto computational frameworks suitable for machine learning can accelerate materials discovery. Combining proposed crystal site feature embedding (CSFE) representation with convolutional and extensive deep neural networks, we achieve a low mean absolute test error of 3.7 meV/atom and 0.069 eV on density functional theory energies and band gaps of mixed halide perovskites. We explore how a small amount of cadmium doping can potentially be applied in solar cell design and sample the large chemical space by using a variational autoencoder to discover interesting perovskites with band gaps in the ultraviolet and infrared. Additionally, we use CSFE to explore chemical spaces and small doping concentrations beyond those used for training. We further show that CSFE has a mean absolute test error of 7 meV/atom and 0.13 eV for total energies and band gaps for 2D perovskites and discuss its adaptability for exploration of an even wider variety of chemical systems.
ChemOS: An orchestration software to democratize autonomous discovery
L. M. Roch, F. Häse, C. Kreisbeck, T. Tamayo-Mendoza, L. P. E. Yunker, J. E. Hein, A. Aspuru-Guzik
Link to Publication Click for AbstractThe current Edisonian approach to discovery requires up to two decades of fundamental and applied research for materials technologies to reach the market. Such a slow and capital-intensive turnaround calls for disruptive strategies to expedite innovation. Self-driving laboratories have the potential to provide the means to revolutionize experimentation by empowering automation with artificial intelligence to enable autonomous discovery. However, the lack of adequate software solutions significantly impedes the development of self-driving laboratories. In this paper, we make progress towards addressing this challenge, and we propose and develop an implementation of ChemOS; a portable, modular and versatile software package which supplies the structured layers necessary for the deployment and operation of self-driving laboratories. ChemOS facilitates the integration of automated equipment, and it enables remote control of automated laboratories. ChemOS can operate at various degrees of autonomy; from fully unsupervised experimentation to actively including inputs and feedbacks from researchers into the experimentation loop. The flexibility of ChemOS provides a broad range of functionality as demonstrated on five applications, which were executed on different automated equipment, highlighting various aspects of the software package.
Machine-learning-accelerated perovskite crystallization
J. Kirman, A. Johnston, D. A. Kuntz, M. Askerka. Y. Gao, P. Todorovic, D. Ma, G. G. Prive, E. H. Sargent
Link to Publication Click for AbstractPerovskites have seen significant research interest in the last decade. As ternary and quaternary compounds, their chemical space is exceptionally large, yet perovskite development has been limited to a restricted set of chemical constituents often discovered through trial and error. Here, we report a high-throughput experimental framework for the discovery of new perovskite single crystals. We use machine learning (ML) to guide the sequence of ever-improved robotic synthetic trials. We perform high-throughput syntheses of perovskite single crystals with a protein crystallization robot and characterize the outcomes with the aid of convolutional neural network-based image recognition. We then use an ML model to predict the optimal conditions for the synthesis of a new perovskite single crystal, enabling us to report the first synthesis of (3-PLA)2PbCl4.This material exhibits strong blue emission, illustrating the applicability of the method in identifying new optoelectronic materials.
Machine learning accelerates discovery of optimal colloidal Quantum dot synthesis
O. Voznyy, L. Levina, J. Fan, M. Askerka, A. Jain, M-J. Choi, O. Ouellette, P. Todorovic, L.K. Sagar, E.H. Sargent
Link to Publication Click for AbstractColloidal quantum dots (CQDs) allow broad tuning of the bandgap across the visible and near-infrared spectral regions. Recent advances in applying CQDs in light sensing, photovoltaics, and light emission have heightened interest in achieving further synthetic improvements. In particular, improving monodispersity remains a key priority in order to improve solar cells’ open-circuit voltage, decrease lasing thresholds, and improve photodetectors’ noise-equivalent power. Here we utilize machine-learning-in-the-loop to learn from available experimental data, propose experimental parameters to try, and, ultimately, point to regions of synthetic parameter space that will enable record-monodispersity PbS quantum dots. The resultant studies reveal that adding a growth-slowing precursor (oleylamine) allows nucleation to prevail over growth, a strategy that enables record-large-bandgap (611 nm exciton) PbS nanoparticles with a well-defined excitonic absorption peak (half-width at half-maximum (hwhm) of 145 meV). At longer wavelengths, we also achieve improved monodispersity, with an hwhm of 55 meV at 950 nm and 24 meV at 1500 nm, compared to the best published to date values of 75 and 26 meV, respectively.